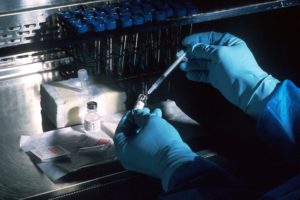
How will we benefit from being able to accurately predict the peptide antigens that the immune system can recognize? Vaccine development would be a major beneficiary of such knowledge (Figure 1).
We could produce more effective and safer vaccines. We could develop vaccines for pathogens for which no vaccine is currently available. We could ensure that vaccines were effective across the genetic diversity of the world’s population. We could develop individualized vaccines based on the naturally occurring polymorphisms in the genes of the immune system that encode the proteins bind and recognize these peptide antigens. We could identify the antigens produced by cancer cells and develop vaccines against those cancers so that they do not spread and cause death. We could determine the antigens that cause autoimmune disease (self-antigens) and match those to the immune proteins that recognize them. We could ensure that vaccines will not trigger autoimmune responses. We could develop strategies to prevent or treat autoimmune disease.
To predict peptide antigens, first we need to understand how the immune system “sees” these peptides. Antigens that activate T cells are presented to the T cells as part of protein complexes called the major histocompatibility complex (MHC) class I or MHC class II. The proteins that bind the antigens are called class I or class II HLA (human leukocyte antigen) (Figure 2).
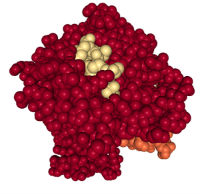
Not only are there multiple members of the class I and class II HLA families, but the HLA genes are highly polymorphic (many naturally occurring genetic variations called alleles). As part of the adaptive immune system, this polymorphism is required so that the immune system can recognize the many different pathogens and diseased cells that people encounter over a lifetime.
This polymorphism creates a technical challenge for antigen identification and prediction, because most cells will have multiple alleles. Thus, matching a specific antigenic peptide to a specific HLA allele is difficult. Abelin and colleagues developed a process to overcome this hurdle. Starting with a cell line that lacked any class I HLA, they used molecular biology to engineer these cells to produce the protein encoded by only one of 16 HLA class I alleles. The antigenic peptides bound by each HLA were identified by mass spectrometry and then bioinformatics and computational biology methods were applied to identify the characteristics of the peptides bound by each of the 16 class I HLA proteins.
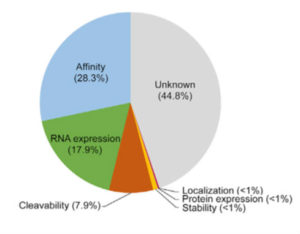
Each HLA protein bound thousands of peptides such that the resulting database contained ~24,000 antigenic peptides, each matched to its partner HLA. With a data set of this large size, the authors identified previously unknown motifs that the HLA proteins recognized and amino acids within the peptides that helped anchor the peptide to the HLA. This large data set also enabled analysis of other factors that contributed to production of antigenic peptides, and identified cleavability of the protein from which the peptide was derived and expression of the gene encoding the protein cleaved to produce the antigenic peptide as two key factors. With this information, the authors developed a prediction tool for each of the 16 class I HLA proteins that included affinity and transcript (gene expression) data. The authors concluded that this tool would double the number of antigens that could be identified for the development of a vaccine.
Although the work of Abelin and colleagues improves antigenic peptide prediction for specific HLA proteins, more work is needed. Similar analysis needs to be done for the other proteins encoded by class I HLA alleles. To replicate this workflow for the analysis of class II HLA proteins, cells deficient in all class II HLA genes need to be engineered to express individual class II HLA genes. Additionally, close to half of the rules governing peptide presentation remain unknown (Figure 3). Affinity of the peptide for the HLA protein, RNA expression of the gene encoding the protein containing the peptide antigen, and cleavability of the protein from which the peptide is derived account for only ~54% of the predictive power of the approach used by Abelin and colleagues. Thus, learning all of the rules that contribute to peptide presentation by HLA proteins will undoubtedly improve the predictive power of future approaches.
Featured Article
J. G. Abelin, D. B. Keskin, S. Sarkizova, C. R. Hartigan, W. Zhang, J. Sidney, J. Stevens, W. Lane, G. L. Zhange, T. M. Eisenhaure, K. R. Clauser, N. Hacohen, M. S. Rooney, S. A. Carr, C. J. Wu, Mass spectrometry profiling of HLA-associated peptidomes in mon-allelic cells enables more accurate epitope prediction. Immunity 46, 315-326 (2017). PubMed
Featured Structure
Cite as: N. R. Gough, Proteomics improves antigen prediction. BioSerendipity (14 June 2017) https://www.bioserendipity.com/2017/06/14/proteomics-improves-antigen-prediction/.